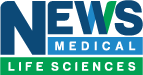
Using data science to make blood banks more efficient
The Sanquin blood bank gathers data on every donation. Around 720,000 donations are made every year. 'That generates a mountain of highly valuable data,' says Leiden PhD candidate Marieke Vinkenoog.
To be able to extract useful information from the data, Sanquin has joined Leiden University's Data Science Research Programme, an interfaculty research program combining specialist knowledge with data science.
'The aim of my PhD research is to use data science to make blood banks more efficient,' explains Vinkenoog, who has been working with Sanquin for the past year via the Data Science Research Programme. 'At the moment I'm looking at the measurements of the hemoglobin levels of donors.' These levels are measured prior to each donation.
Hemoglobin levels
There has to be a sufficient level of the protein hemoglobin in the blood to transport oxygen throughout the body. When a donor gives blood, about half a liter of blood is taken, containing hemoglobin. If the hemoglobin level is too low, it could mean that the donor is left with too low a level of hemoglobin after giving blood. Donating blood would then be bad for the donor's health, and they would not be able to give blood on that occasion. They can return after three months to try again.
People who are unable to donate blood for this reason often don't come back to the blood bank after being refused two or three times. This means that Sanquin loses donors.
Predictive modeling
A hemoglobin level that is too low to allow a donor to give blood can be caused by diet and lifestyle. After giving blood, it takes a number of weeks before the level is restored. How quickly that happens varies from person to person. Sanquin has therefore been working for a number of years with models that predict how often they can call on donors without causing their hemoglobin levels to fall too low, which could result in a fruitless trip to the blood bank. 'Traditional statistical models were always used,' Vinkenoog explains. These models work best with structured data, where a person's hemoglobin level is measured regularly, possibly weekly. But Sanquin's data come from the real world, where data collection can be rather irregular: sometimes a person will come and give blood again after three months, or maybe after two years. That makes it difficult to construct a predictive model.'
For some loyal donors, who have been giving blood for maybe ten years, there may be no regular measurements, but nonetheless there are a lot of measurements. Vinkenoog also makes use of these data. 'I'm hoping to discover a predictable trend in the data using modern machine learning techniques. These can be trained to recognize relationships in large amounts of data.'
If Vinkenoog develops a model for the hemoglobin level within the coming year, she will be able to explore other ways of personalizing blood donation so that it fits better in the life of the donor. 'But for now I have my hands full with the hemoglobin data.'
Source:
No hay comentarios:
Publicar un comentario