A Diabetic Retinopathy Screening Tool for Low-Income Adults in Mexico
ORIGINAL RESEARCH — Volume 14 — October 12, 2017
Kenny Mendoza-Herrera, MS1; Amado D. Quezada, MS2; Andrea Pedroza-Tobías, MS1; Cesar Hernández-Alcaraz, MS1; Jans Fromow-Guerra, PhD3; Simón Barquera, PhD1 (View author affiliations)
Suggested citation for this article: Mendoza-Herrera K, Quezada AD, Pedroza-Tobías A, Hernández-Alcaraz C, Fromow-Guerra J, Barquera S. A Diabetic Retinopathy Screening Tool for Low-Income Adults in Mexico. Prev Chronic Dis 2017;14:170157. DOI: http://dx.doi.org/10.5888/pcd14.170157.
Abstract
Introduction
A national diabetic retinopathy screening program does not exist in Mexico as of 2017. Our objective was to develop a screening tool based on a predictive model for early detection of diabetic retinopathy in a low-income population.
Methods
We analyzed biochemical, clinical, anthropometric, and sociodemographic information from 1,000 adults with diabetes in low-income communities in Mexico (from 11,468 adults recruited in 2014–2016). A comprehensive ophthalmologic evaluation was performed. We developed the screening tool through the following stages: 1) development of a theoretical predictive model, 2) performance assessment and validation of the model using cross-validation and the area under the receiver operating characteristic curve (AUC ROC), and 3) optimization of cut points for the classification of diabetic retinopathy. We identified points along the AUC ROC that minimized the misclassification cost function and considered various scenarios of misclassification costs and diabetic retinopathy prevalence.
Results
Time since diabetes diagnosis, high blood glucose levels, systolic hypertension, and physical inactivity were considered risk factors in our screening tool. The mean AUC ROC of our model was 0.780 (validation data set). The optimized cut point that best represented our study population (z = −0.640) had a sensitivity of 82.9% and a specificity of 61.9%.
Conclusion
We developed a low-cost and easy-to-apply screening tool to detect people at high risk of diabetic retinopathy in Mexico. Although classification performance of our tool was acceptable (AUC ROC > 0.75), error rates (precision) depend on false-negative and false-positive rates. Therefore, confirmatory assessment of all cases is mandatory.
Introduction
In 2016, diabetes was declared a national epidemiologic emergency in Mexico (1). In 2006, the estimated prevalence of diabetes in Mexican adults was 14.4% (2). Mortality rates attributable to this disease in Mexico are among the highest in the world (3). By 2012, 74.7% of Mexican adults with diagnosed diabetes had inadequate glycemic control (4). Diabetes is associated with the development and progression of diabetic retinopathy (5–8), a major cause of sight loss and blindness in Latin American countries (9). A population-based survey from 2010 in the state of Chiapas found that 38.9% of adults aged 50 or older with diabetes had diabetic retinopathy and 21.0% had proliferative diabetic retinopathy (10).
Long-term diabetes and hypertension are consistently associated with diabetic retinopathy (5–8,11–13). The Mexican National Nutrition Survey 2006 found that the mean time since diabetes diagnosis among adults was more than 8 years (2). In 2012, an estimated 65.6% of adults with diabetes had hypertension (14). In this context, an epidemic of diabetes complications, including diabetic retinopathy, could worsen in Mexico, and the study of screening systems for diabetic retinopathy is important.
Diabetic retinopathy ranks third in direct costs generated by diabetes complications in Mexico (15); these costs result from specialized procedures for diagnosis and treatment. A cost-benefit analysis to identify optimal cut points for identifying people who are at risk for diabetic retinopathy and who need a comprehensive ophthalmologic evaluation is an approach to developing an adequate-performance screening tool (16); however, such an approach would be complex because of the detailed cost information required.
Our objective was to develop a practical screening tool based on a predictive model and a simplification of a cost-benefit analysis to optimize cut points for early detection of diabetic retinopathy in low-income communities in Mexico.
Methods
We conducted a screening protocol for eye-related complications of diabetes from May 1, 2014, to June 30, 2016, in 3 low-income municipalities in the state of Morelos. We recruited 11,468 adults (aged ≥20 y) for a screening of chronic diseases in our mobile unit and community health centers. From these participants, we invited those with a type 2 diabetes diagnosis (n = 1,768 [15.4%]) to a comprehensive ophthalmologic evaluation. Exclusion criteria for this evaluation were signs of ocular infection or pregnancy.
Of the 1,768 participants, 538 declined to participate in the ophthalmologic evaluation, 1 person was excluded because the quality of photographs was not adequate for grading, and 229 participants did not have a photographic assessment at the time of analysis. One thousand participants (56.6%) completed the procedure. We obtained informed consent from all participants, and the protocol was approved by the ethics, research, and biosecurity committees of the Mexican National Institute of Public Health.
Data collection and definition of variables
All participants had at least 1 glycemic assessment (fasting [≥8 h] capillary or random capillary glycemia [glucometer method] or fasting venous glycemia [glucose oxidase method]). Fasting serum triglycerides, total cholesterol, and high-density lipoprotein cholesterol were assessed by enzymatic method (n = 418) and serum insulin with radioimmunoassay method (n = 112) for a portion of the sample; because of logistical and budgetary constraints, the entire sample could not be assessed for these variables.
High blood glucose was defined as a fasting glucose of 126 mg/dL or more or, if fasting glucose was unavailable, as random glucose of 200 mg/dL or more (17). Insulin resistance was classified by using a homeostasis model assessment value of 3.8 or more (18).
Hypertriglyceridemia was defined as triglycerides of 150 mg/dL or more, hypercholesterolemia as total cholesterol of 200 mg/dL or more, and hypoalphalipoproteinemia as high-density lipoprotein cholesterol of less than 50 mg/dL for women and less than 40 mg/dL for men (19,20).
Blood pressure was measured twice (interval of 30 seconds). We diagnosed high systolic/diastolic blood pressure when the average of the assessments was ≥140/≥90 mm Hg. Likewise, we recorded whether participants reported a diagnosis of hypertension (21).
Weight, height, and waist circumference were measured by trained personnel using standard protocols. Body mass index (BMI; weight in kilograms divided by height in m2 [kg/m2]) was calculated: overweight was defined as a BMI of 25.0 to 29.9 and obesity as a BMI of 30.0 or more (22). Abdominal obesity was defined as a waist circumference of 80 cm or more for women and 90 cm or more for men (19).
Data on sociodemographic characteristics and clinical history were collected by trained interviewers through an adapted version of the questionnaires applied in the National Health and Nutrition Survey of Mexico (23). We used the time since diabetes diagnosis as a proxy of duration of type 2 diabetes and categorized it into 4 intervals (<5 y, 5 y to <10 y, 10 y to <15 y and ≥15 y). Participants reported whether they followed diet and physical activity recommendations to control their diabetes.
We conducted a principal component analysis of 15 characteristics related to household appliances and services (eg, ownership of car, telephone, computer, vacuum cleaner, washing machine, refrigerator, pay television, internet) as a proxy for socioeconomic status (SES). Similar methods have been used (14). These characteristics had a factorial loading of 0.30 or more. The first principal component was divided into tertiles and used as a proxy for low SES, medium SES, and high SES.
Ophthalmologic evaluation
All participants were interviewed by using a validated questionnaire for ocular assessment. The following data were collected by trained technicians: best-corrected visual acuity, refractometry (by using an automated refractor [Huvitz HRK-7000]), and intraocular pressure (by using a rebound tonometer [Icare TA01i]). Afterwards, all participants received a photographic evaluation of their posterior pole (45° nonmydriatic fundus camera [DRS-Centervue]). Participants were dilated with tropicamide only if the quality of the photographs was not adequate for grading. We took 3 fields of the posterior pole using a standardized protocol. The first field centered on the optic nerve, the second field centered on the fovea, and third field was temporal to the macula but included the fovea. This protocol has an adequate level of sensitivity and specificity for grading referable stages of diabetic retinopathy (24).
All photographs were sent to Eye Knowledge Network (www.eyeknowledge.net). All cases were masked and reviewed by trained graders from the Hospital Luis Sánchez Bulnes of the Association for the Prevention of Blindness in Mexico. The cases were graded by using the Revised English Diabetic Eye Screening Program Grading System (25), which allows prompt referral of proliferative stages of diabetic retinopathy and macular edema. Diabetic retinopathy was recorded when a participant had background diabetic retinopathy, preproliferative diabetic retinopathy, or proliferative diabetic retinopathy.
Statistical analysis
We tabulated categorical variables as frequency and proportion distributions and quantitative variables as measures of central tendency (mean or median) and dispersion (standard deviation [SD] or interquartile range). We set statistical significance at an α of .05. We compared measures of central tendency according to diabetic retinopathy status of participants (has diabetic retinopathy or does not have diabetic retinopathy) by using the Student t test or Mann–Whitney U test, depending on the distribution of the quantitative variables. We used a χ2 test or Fisher exact test to compare the prevalence of diabetic retinopathy across categories of nonquantitative variables. We conducted a descriptive analysis to compare sociodemographic and clinical characteristics and diabetic retinopathy risk factors between participants and nonparticipants.
We developed the screening tool in 3 stages: 1) we developed the theoretical predictive model, 2) we assessed the performance of the model and conducted a validation analysis, and 3) we optimized risk-score cut points for diabetic retinopathy classification.
Development of the theoretical predictive model
For multivariate analysis, we included only participants who had complete information on diabetic retinopathy status (the dependent variable), and we determined whether at least 95% of the participants provided information for each of the independent variables. If 5% or more of the participants did not provide information for an independent variable (theoretical risk factors of diabetic retinopathy), we used multiple imputation through a logistic regression model, where diabetic retinopathy, sex, age, and self-reported diabetes screening were the independent variables, to complete the information.
We generated a predictive probit model based on theoretical risk factors of diabetic retinopathy (5–8,11–13). We decided to use this model to develop our tool because of its easy interpretability as a z score from its linear equation and because it provides a predicted probability for the linear predictor (applying the standard normal cumulative function). Familiarity with this distribution provides a better understanding of coefficients and predicted z scores. The dependent variable was diabetic retinopathy, and the 4 predictors were time since diabetes diagnosis, high blood glucose, high systolic blood pressure, and physical inactivity. We estimated probabilities adjusted by covariables of having diabetic retinopathy given each risk factor category though predictive margins.
Performance assessment and validation
We used the k-fold cross-validation method (k = 10 partitions) and the area under the receiver operating characteristic curve (AUC ROC). To assess the performance of the model in training and validation data sets, we randomly divided the sample into 10 partitions. In each partition, one segment was reserved for model validation (validation data set, n ~ 10%), while the rest of the sample in this partition was used as a training subsample (training data set, n ~ 90%). We calculated the AUC ROC for each iteration and its mean for the 10 iterations.
Optimization of risk-score cut points for diabetic retinopathy classification
We developed a risk score for diabetic retinopathy based on the z predictor of our statistical model. In this way, the attributable score of each risk factor was equivalent to its probit coefficient.
The use of a cost-benefit analysis to select cut points implies knowledge of true and false classification costs; however, it is difficult to have such complete information. To select the optimal cut points of the z predictor to classify diabetic retinopathy, we decided to focus on misclassification costs only through the misclassification cost term (16). We identified points along the ROC curve that minimized the misclassification cost function for various scenarios of misclassification costs and diabetic retinopathy prevalence. The costs of true classification were assumed as null, and the examples of the variations of misclassification ratios were set according to consequences in health costs of screening for diabetic retinopathy.
We estimated sensitivity and specificity across AUC ROC and isocost curves, which minimized the costs of misclassification. Likewise, we estimated positive predictive values and negative predictive values.
We considered the following scenarios for the optimization of the cut points: diabetic retinopathy prevalence of 35.0%, 40.0%, and 45.0%, and the observed prevalence in our sample. We examined various ratios of cost misclassification (classification costs of false negatives divided by classification costs of false positives). We examined ratios of 1, 4, and 10, assuming that classification of a false negative would generate higher health care costs than would classification of a false positive.
The statistical analysis was conducted by using Stata version 13.1 (StataCorp LLC) and RStudio version 1.0.136 with the OptimalCutpoints package.
Results
The mean age of our sample was 57.2 y (SD, 11.0 y), and 73.0% were women. The prevalence of diabetic retinopathy was 31.7% (Table 1); 18.9% had background diabetic retinopathy, 5.7% had preproliferative diabetic retinopathy, and 7.1% of participants had active proliferative diabetic retinopathy.
The prevalence of diabetic retinopathy was significantly higher among participants with insulin resistance, high blood glucose, and hypertension than among participants without those conditions. Participants with diabetic retinopathy had significantly longer times since diabetes diagnosis, higher blood glucose levels, and higher systolic blood pressure than those without diabetic retinopathy. In contrast, the prevalence of diabetic retinopathy was lower among participants who were overweight or obese, had abdominal obesity, or used physical activity to control their diabetes than among participants without these characteristics. The prevalence of diabetic retinopathy was highest, by SES, in the lowest tertile of SES and highest, by marital status, among divorced adults (Table 1).
We found no significant differences in the distribution of sociodemographic characteristics, clinical characteristics, or diabetic retinopathy risk factors between participants and nonparticipants.
Development and cross-validation of predictive model
From all independent variables included in our model, except physical activity (data were missing for 17.0% of participants), had at least 95.0% of information. After multiple imputation analysis for physical activity, we obtained a probit model with 939 observations.
According to our multivariate analysis (Table 2), time since diabetes diagnosis was positively associated with the estimated probability of diabetic retinopathy. For example, the probability of diabetic retinopathy was 11.4% (95% confidence interval [CI], 7.9%–14.9%) when time since diabetes diagnosis was less than 5 years, whereas the probability was 56.0% (95% CI, 49.5%–62.6%) when time since diabetes diagnosis was 15 years or more. Similarly, the probability of diabetic retinopathy was higher among those with high blood glucose (35.6%) and high systolic blood pressure (37.4%) than among those without those conditions (23.9% and 29.3%, respectively). On the other hand, participants who reported using physical activity to control diabetes had a lower predicted probability of diabetic retinopathy (25.4%) than those who reported not using physical activity (34.8%).
According to the cross-validation analysis (Table 3), the diagnostic performance of our model was similar between training data sets (mean AUC ROC = 0.780) and validation data sets (mean AUC ROC = 0.778).
Risk-score cut points for diabetic retinopathy classification
According to the prevalence of diabetic retinopathy observed with misclassification ratios of 1, 4, and 10, the optimal cut points were −0.046, −0.640, and −1.209, respectively (Table 4).
Four points minimized the misclassification costs given the ROC curve of our model (Figure). The optimized cut point according to a misclassification ratio of 4 and the diabetic retinopathy prevalence observed in our sample (31.7%) was z = −0.640, with a sensitivity of 82.9%, a specificity of 61.9%, a positive predictive value of 50.3%, and a negative predictive value of 88.6% (Table 4).
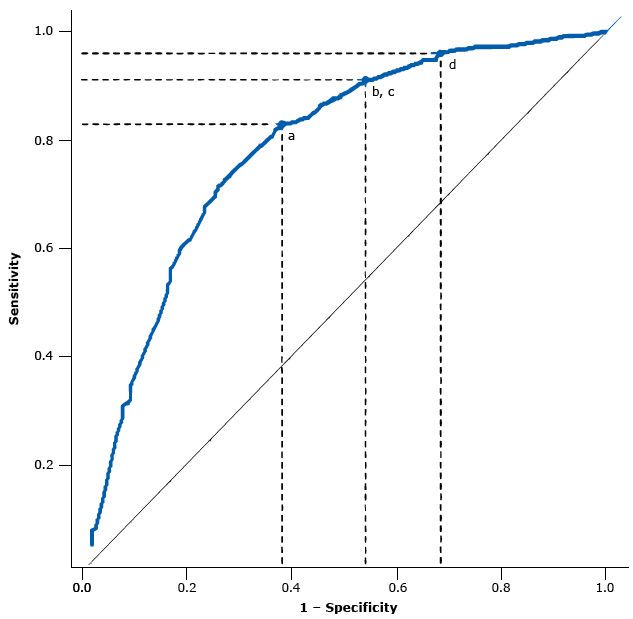
Figure.
Area under the receiver operating characteristic (ROC) curve and points along the ROC curve corresponding to optimized cut points given a cost ratio (classification costs of false negatives divided by classification costs of false positives) equal to 4 and various scenarios of diabetic retinopathy prevalence: a) 31.7%, the observed prevalence in the study population; b) and c) prevalence of 35.0% and 40.0%; and d) prevalence of 45.0%. [A tabular description of this figure is also available.]
Area under the receiver operating characteristic (ROC) curve and points along the ROC curve corresponding to optimized cut points given a cost ratio (classification costs of false negatives divided by classification costs of false positives) equal to 4 and various scenarios of diabetic retinopathy prevalence: a) 31.7%, the observed prevalence in the study population; b) and c) prevalence of 35.0% and 40.0%; and d) prevalence of 45.0%. [A tabular description of this figure is also available.]
On the basis of our data, we propose a risk-score screening tool (Box): A health care provider (can be a nonspecialized provider) asks the patient 2 questions (on time since diabetes diagnosis and use of physical activity to control diabetes) and obtains 2 measurements (blood glucose and systolic blood pressure). Each response is scored, the scores are summed, and a final score is calculated. The health care provider consults a simple chart that shows 4 levels of diabetic retinopathy prevalence, chooses the prevalence that most closely matches the prevalence of the community in which the patient resides, and then identifies the cut point that corresponds with the prevalence. If the patient has a score equal to or greater than the cut point, the patient should be directed to receive a comprehensive ophthalmologic evaluation.
Discussion
We developed a practical screening tool for diabetic retinopathy that could be used by nonspecialized health care personnel in low-income settings. The tool requires information on 4 risk factors. Other risk scores exist (26,27); unlike these, we optimized various cut points according to misclassification costs and diabetic retinopathy prevalence. This optimization allows the application of this tool in various contexts.
We assumed that classifying people as not having diabetic retinopathy when they actually have the condition (false negative) would result in higher long-term health care costs than would classifying them with the disease when they do not have it (false positive), because without timely diagnosis and treatment, these people are likely to progress to advanced stages of the condition. We recommend using the cut points for misclassification ratios of 4 and 10, which gives greater importance to sensitivity than to specificity. Although this recommendation substantially decreases specificity, it does not imply negative health effects, because all people with type 2 diabetes should receive an ophthalmologic evaluation when diabetes is diagnosed (17).
Although the rate of false positives generated by our tool could increase health care costs (as a result of comprehensive ophthalmologic evaluations), the application of our tool could help improve compliance with recommendations for obtaining these evaluations. In addition, the benefits of timely diagnosis and treatment could compensate for any increases in health care costs.
Although we did not have complete information for a cost-benefit analysis, we showed how results changed when the relative importance of the cost of false negatives (type 2 error) to the cost of false positives (type 1 error) varied. We set false-negative rates to be higher than false-positive rates because the health care costs resulting from delays in diagnosis and treatment of false negatives may be high in the context of the screening of diabetic retinopathy. Although the classification performance of our tool was acceptable (AUC ROC > 0.75), the precision of classification depends on the false-negative rate and false-positive rate. Therefore, confirmatory assessment of all cases is mandatory. Additionally, the negative cases identified by this tool also are at some risk of diabetic retinopathy, so periodic exploratory evaluations should be performed in all patients with diabetes.
We presented misclassification ratios only as examples: different ratios could be assumed for future research or in different contexts. Our study demonstrated a simplified approach for developing a screening tool based on a misclassification-cost criterion. Future research should focus on the assignment of costs for the 4 classification types (true positives, true negatives, false positives, and false negatives) on diabetic retinopathy screening context.
We found that systolic blood pressure and the lack of physical activity were associated with diabetic retinopathy; some studies showed that high systolic blood pressure is a potentially modifiable risk factor for diabetic retinopathy (7,12). Physical inactivity could be another important modifiable risk factor for diabetic retinopathy because it is associated with poor glycemic control (28). Our study showed that a simple question about physical activity can predict a significantly lower probability of diabetic retinopathy. Although the question cannot determine whether a person is implementing this lifestyle recommendation, it may reflect awareness and knowledge of self-care practices.
Consistent with other researchers (29,30), we observed a negative effect of obesity on diabetic retinopathy. Participants with overweight and obesity had lower levels of blood glucose and less time since diabetes diagnosis than did underweight and normal-weight participants (data not shown). We believe that the negative effect of obesity on diabetic retinopathy may be attributed to the fact that people with excess weight are experiencing an earlier stage of diabetes than people with normal or low weight.
We found a higher proportion of women (73.0%) than men in our study sample possibly because women engage in self-care practices and informal unpaid activities more than men do; this engagement may have facilitated their attendance to the recruitment process. We found a lower systolic blood pressure among women than among men (data not shown), which, given the higher proportion of women, could have underestimated the effect of systolic blood pressure in our analysis.
Our study has limitations. We did not measure HbA1c, which prevented us from adjusting our model by a variable of long-term glycemic control. However, our model adequately predicted diabetic retinopathy using parameters that are easier to measure and less expensive than an HbA1c test, which is not available at all primary health care service locations in Mexico.
An important portion of the population with type 2 diabetes may not receive a diagnosis for years (17). In Mexico, almost half of the population with diabetes is not diagnosed during routine health care, and many of them have complications that indicate many years of living with the disease (2). However, it was not possible to assess how long our study participants had been living with diabetes. Because the onset of type 2 diabetes can occur at any point during adulthood (random error), age is not the best indicator of diabetes duration. Instead of age, we used time since diagnosis as a variable for diabetes duration. Self-report of time since diabetes diagnosis may underestimate duration, but we considered it to be a nondifferential systematic error that did not affect our results. People with type 2 diabetes may recall onset of their disease inaccurately, but the inaccuracy is the same across the population of people with diabetes, and recall of onset is independent of the diabetic retinopathy condition.
The high health cost of diabetic retinopathy in Mexico is due in part to the lack of a program designed to prevent diabetes complications (15). A challenge for our team will be to develop pilot studies that evaluate the feasibility, functionality, and costs of offering our screening tool at primary health care service locations as a strategy for strengthening the system for ophthalmologic evaluation of people with diabetes.
Early detection strategies must be implemented to reduce the burden of diabetic retinopathy. Our new screening tool is a promising approach and a practical strategy with an adequate performance to detect risk of diabetic retinopathy in adults with type 2 diabetes in low-income communities in Mexico.
Acknowledgments
The authors thank Luisa Torres-Sanchez, Selene Pacheco-Miranda, and Hector Lamadrid-Figueroa and ophthalmologists Raul Velez-Montoya and Gerardo García-Aguirre. This study was funded by the World Diabetes Foundation (WDF-13788), by a scholarship from the Mexican Council of Science and Technology (Spanish acronym: CONACYT), and by National Institutes of Health–Fogarty RO3 project no. TW009061. The authors declare no financial or personal conflict of interest.
Author Information
Corresponding Author: Simón Barquera, PhD, Instituto Nacional de Salud Pública, Cuernavaca, Morelos, México, Avenida Universidad #655 Col. Sta. Ma. Ahuacatitlán, CP 62100. Telephone: 52-777-329-30-17. Email: sbarquera@insp.mx.
Author Affiliations: 1Center for Nutrition and Health Research, National Institute of Public Health, Cuernavaca, Morelos, México. 2Center for Evaluation and Surveys Research, National Institute of Public Health, Cuernavaca, Morelos, México. 3Association for the Prevention of Blindness in Mexico, México City, México.
References
- Mexican Ministry of Health. Epidemiological emergency statement EE-4-2016 for all the states of Mexico due to the magnitude and transcendence of diabetes mellitus cases, November 1, 2016. Mexico City (MX): Mexican National Center for Preventive Programs and Disease Control (CENAPRECE); 2016. http://www.cenaprece.salud.gob.mx/programas/interior/emergencias/descargas/pdf/EE_4.pdf. Accessed August 23, 2017.
- Villalpando S, de la Cruz V, Rojas R, Shamah-Levy T, Avila MA, Gaona B, et al. Prevalence and distribution of type 2 diabetes mellitus in Mexican adult population: a probabilistic survey. Salud Publica Mex 2010;52(Suppl 1):S19–26. CrossRef PubMed
- Barquera S, Pedroza-Tobias A, Medina C. Cardiovascular diseases in mega-countries: the challenges of the nutrition, physical activity and epidemiologic transitions, and the double burden of disease. Curr Opin Lipidol 2016;27(4):329–44. CrossRef PubMed
- Jiménez-Corona A, Aguilar-Salinas CA, Rojas-Martínez R, Hernández-Ávila M. Type 2 diabetes and frequency of prevention and control measures. Salud Publica Mex 2013;55(Suppl 2):S137–43. PubMed
- Kim YJ, Kim JG, Lee JY, Lee KS, Joe SG, Park JY, et al. Development and progression of diabetic retinopathy and associated risk factors in Korean patients with type 2 diabetes: the experience of a tertiary center. J Korean Med Sci 2014;29(12):1699–705. CrossRef PubMed
- Mohamed Q, Gillies MC, Wong TY. Management of diabetic retinopathy: a systematic review. JAMA 2007;298(8):902–16. CrossRef PubMed
- Liu Y, Wang M, Morris AD, Doney AS, Leese GP, Pearson ER, et al. Glycemic exposure and blood pressure influencing progression and remission of diabetic retinopathy: a longitudinal cohort study in GoDARTS. Diabetes Care 2013;36(12):3979–84. CrossRef PubMed
- Klein R, Klein BE, Moss SE, Cruickshanks KJ. The Wisconsin Epidemiologic Study of Diabetic Retinopathy: XVII. The 14-year incidence and progression of diabetic retinopathy and associated risk factors in type 1 diabetes. Ophthalmology 1998;105(10):1801–15. CrossRef PubMed
- Limburg H, Espinoza R, Lansingh VC, Silva JC. Functional low vision in adults from Latin America: findings from population-based surveys in 15 countries. Rev Panam Salud Publica 2015;37(6):371–8. PubMed
- Polack S, Yorston D, López-Ramos A, Lepe-Orta S, Baia RM, Alves L, et al. Rapid assessment of avoidable blindness and diabetic retinopathy in Chiapas, Mexico. Ophthalmology 2012;119(5):1033–40. CrossRef PubMed
- Matthews DR, Stratton IM, Aldington SJ, Holman RR, Kohner EM; UK Prospective Diabetes Study Group. Risks of progression of retinopathy and vision loss related to tight blood pressure control in type 2 diabetes mellitus: UKPDS 69. Arch Ophthalmol 2004;122(11):1631–40. CrossRef PubMed
- Klein BE, Klein R, Moss SE, Palta M. A cohort study of the relationship of diabetic retinopathy to blood pressure. Arch Ophthalmol 1995;113(5):601–6. CrossRef PubMed
- Yau JW, Rogers SL, Kawasaki R, Lamoureux EL, Kowalski JW, Bek T, et al. Global prevalence and major risk factors of diabetic retinopathy. Diabetes Care 2012;35(3):556–64. CrossRefPubMed
- Campos-Nonato I, Hernández-Barrera L, Rojas-Martínez R, Pedroza A, Medina-García C, Barquera-Cervera S. [Hypertension: prevalence, early diagnosis, control and trends in Mexican adults]. Salud Publica Mex 2013;55(Suppl 2):S144–50. CrossRef PubMed
- Barquera S, Campos-Nonato I, Aguilar-Salinas C, Lopez-Ridaura R, Arredondo A, Rivera-Dommarco J. Diabetes in Mexico: cost and management of diabetes and its complications and challenges for health policy. Global Health 2013;9(1):3. CrossRef PubMed
- López-Ratón M, Rodriguez-Álvarez MX, Cardaso-Suárez C, Gude-Sampedro F. OptimalCutpoints: an R package for selecting optimal cutpoints in diagnostic tests. J Stat Softw 2014;61(8).CrossRef
- American Diabetes Association. 2. Classification and diagnosis of diabetes. Diabetes Care 2017;40(Suppl 1):S11–24. CrossRef PubMed
- Qu HQ, Li Q, Rentfro AR, Fisher-Hoch SP, McCormick JB. The definition of insulin resistance using HOMA-IR for Americans of Mexican descent using machine learning. PLoS One 2011;6(6):e21041. CrossRef PubMed
- Alberti KG, Eckel RH, Grundy SM, Zimmet PZ, Cleeman JI, Donato KA, et al. Harmonizing the metabolic syndrome: a joint interim statement of the International Diabetes Federation Task Force on Epidemiology and Prevention; National Heart, Lung, and Blood Institute; American Heart Association; World Heart Federation; International Atherosclerosis Society; and International Association for the Study of Obesity. Circulation 2009;120(16):1640–5. CrossRef PubMed
- National Cholesterol Education Program (NCEP) Expert Panel on Detection, Evaluation, and Treatment of High Blood Cholesterol in Adults (Adult Treatment Panel III). Third Report of the National Cholesterol Education Program (NCEP) Expert Panel on Detection, Evaluation, and Treatment of High Blood Cholesterol in Adults (Adult Treatment Panel III) final report. Circulation 2002;106(25):3143–421. PubMed
- Aronow WS, Fleg JL, Pepine CJ, Artinian NT, Bakris G, Brown AS, et al. ACCF/AHA 2011 expert consensus document on hypertension in the elderly: a report of the American College of Cardiology Foundation Task Force on Clinical Expert Consensus documents developed in collaboration with the American Academy of Neurology, American Geriatrics Society, American Society for Preventive Cardiology, American Society of Hypertension, American Society of Nephrology, Association of Black Cardiologists, and European Society of Hypertension. J Am Coll Cardiol 2011;57(20):2037–114. CrossRef PubMed
- World Health Organization. Obesity: preventing and managing the global epidemic. Report of a WHO consultation. Geneva (CH): World Health Organization; 2000. Technical report series no. 894.
- Olaiz G, Rivera-Dommarco JA, Shamah-Levy T, Rojas-Martínez R, Villalpando S, Hernández-Ávila M: Encuesta Nacional de Salud y Nutrición 2006. Cuernavaca (Morelos): 2006.
- Vujosevic S, Benetti E, Massignan F, Pilotto E, Varano M, Cavarzeran F, et al. Screening for diabetic retinopathy: 1 and 3 nonmydriatic 45-degree digital fundus photographs vs 7 standard early treatment diabetic retinopathy study fields. Am J Ophthalmol 2009;148(1):111–8. CrossRef PubMed
- Broadbent D. Diabetic retinopathy: fundamentals for primary care. Diabetes & Primary Care 2013;15(4):201–10.
- Wang J, Chen H, Zhang H, Yang F, Chen RP, Li YB, et al. The performance of a diabetic retinopathy risk score for screening for diabetic retinopathy in Chinese overweight/obese patients with type 2 diabetes mellitus. Ann Med 2014;46(6):417–23. CrossRef PubMed
- Azrak C, Palazón-Bru A, Baeza-Díaz MV, Folgado-De la Rosa DM, Hernández-Martínez C, Martínez-Toldos JJ, et al. A predictive screening tool to detect diabetic retinopathy or macular edema in primary health care: construction, validation and implementation on a mobile application. PeerJ 2015;3:e1404. CrossRef PubMed
- Umpierre D, Ribeiro PA, Kramer CK, Leitão CB, Zucatti AT, Azevedo MJ, et al. Physical activity advice only or structured exercise training and association with HbA1c levels in type 2 diabetes: a systematic review and meta-analysis. JAMA 2011;305(17):1790–9. CrossRef PubMed
- Klein R, Klein BE, Moss SE. Is obesity related to microvascular and macrovascular complications in diabetes? The Wisconsin Epidemiologic Study of Diabetic Retinopathy. Arch Intern Med 1997;157(6):650–6. CrossRef PubMed
- Klein R, Klein BE, Moss SE, Davis MD, DeMets DL. The Wisconsin epidemiologic study of diabetic retinopathy. III. Prevalence and risk of diabetic retinopathy when age at diagnosis is 30 or more years. Arch Ophthalmol 1984;102(4):527–32. CrossRef PubMed
No hay comentarios:
Publicar un comentario