Sub-County Life Expectancy: A Tool to Improve Community Health and Advance Health Equity
TOOLS AND TECHNIQUES — Volume 15 — January 25, 2018
Vickie L. Boothe, MPH1; Leslie A. Fierro, PhD, MPH2; Amy Laurent, MSPH3; Margaret Shih, PhD4 (View author affiliations)
Suggested citation for this article: Boothe VL, Fierro LA, Laurent A, Shih M. Sub-County Life Expectancy: A Tool to Improve Community Health and Advance Health Equity. Prev Chronic Dis 2018;15:170187. DOI: http://dx.doi.org/10.5888/pcd15.170187.
PEER REVIEWED
On This Page
- Abstract
- Need for Sub-County Population Health Indicators
- Advantages of Life Expectancy at Birth Compared with Other Local Measures
- Demonstrated Utility of Local Life Expectancy at Birth
- Resources for Calculating Sub-County Life Expectancy at Birth
- SCALE Phase 1 Results and Lessons Learned
- Discussion
- Acknowledgments
- Author Information
- References
- Tables
Abstract
Compared with people in other developed countries, Americans live shorter lives, have more disease and disability, and lag on most population health measures. Recent research suggests that this poor comparative performance is primarily driven by profound local place-based disparities. Several initiatives successfully used sub-county life expectancy estimates to identify geographic disparities, generate widespread interest, and catalyze multisector actions. To explore the feasibility of scaling these efforts, the Centers for Disease Control and Prevention and the Council of State and Territorial Epidemiologists initiated a multiphase project — the Sub-County Assessment of Life Expectancy. Phase I participants reviewed the literature, assessed and identified appropriate tools, calculated locally relevant estimates, and developed methodological guidance. Phase I results suggest that most state and local health departments will be able to calculate actionable sub-county life expectancy estimates despite varying resources, expertise, and population sizes, densities, and geographies. To accelerate widespread scaling, we describe several successful case examples, identify user-friendly validated tools, and provide practical tips that resulted from lessons learned.
Need for Sub-County Population Health Indicators
Safer workplaces, vaccinations, improved motor-vehicle safety, and other twentieth-century public health achievements measurably improved health and increased longevity worldwide (1). In the United States, life expectancy at birth (LE), a key population health measure, increased steadily, reaching an all-time high of 78.8 years in 2012 (2). Since then, however, American LE has stalled. After increasing modestly from 2012 to 2014, LE unexpectedly declined to 78.8 years in 2015, adding to concerns about our nation’s health (2,3). Despite spending more than double on health care than other developed countries, Americans increasingly live shorter lives, experience more disease and disability across the lifespan, and lag on most population health measures (4).
Profound and persistent local geographic disparities are primary drivers of America’s poor performance (5,6). In 2010, LEs for females in Marin County, California (85.02 y), and males in Fairfax County, Virginia (81.67 y), were equivalent to the longest-lived countries of Japan and Switzerland. In contrast, LEs for males in McDowell County, West Virginia (63.90 y), and females in Perry County, Kentucky (72.65 y), were lower than estimates for Bangladesh and Nicaragua (5). Researchers suggest that these disparities are driven by several factors, including health care access; socioeconomic factors; and environmental, behavioral, and physiological risk factors (5).
Addressing America’s poor performance requires a shift in approach, which has focused historically on medical interventions, behaviors, and lifestyle choices (4). Accordingly, public health officials have called for development of locally relevant and timely neighborhood-level health and other indicators to drive actions that address underlying health determinants such as housing, economic development, and environment (7). This latest call to action adds to the growing body of literature documenting an urgent need for community-level health indicators. Without valid, reliable local indicators, health departments are constrained in their ability to detect disparity “hot spots,” identify correlated determinants, and catalyze effective, targeted, multisector actions (8–11).
Advantages of Life Expectancy at Birth Compared with Other Local Measures
Unique mathematical and other properties suggest that local LE is better suited for driving actions than are other mortality measures (12). LE enables direct comparisons across time and geographic areas with diverse population structures and is easier to interpret than standardized mortality ratios or age-adjusted mortality rates (13–15). Stratifying LE by demographic characteristics such as race and income can elucidate disparities, inform resource allocation, and catalyze policy changes (9). LE has greater utility than modeled-based small-area estimates of national and state health survey data, which cannot be used to evaluate intervention effects (16) and can be affected by recall and selection bias (17). Furthermore, several studies document the feasibility of generating robust and accurate LE for small populations. Using Monte Carlo simulations, researchers evaluated methods for generating LE for the United Kingdom’s electoral wards, which in 2001 had a mean population of 5,959 (range, 995–35,770) (11,13,18). The adjusted Chiang II life table method was judged to produce accurate and reliable estimates for populations of 5,000 person-years-at-risk or more with standard errors of approximately 2 years. Because ward-level LE disparities were estimated to exceed 10 years, standard errors of 2 years and associated 95% confidence intervals of approximately 7 years allowed identification of wards with statistically different values (18). Subsequently, researchers evaluating methods for local jurisdictions in New South Wales and in Austria, Italy, Japan, Spain, Sweden, and the United Kingdom confirmed that populations smaller than 5,000 person-years-at-risk yielded biased LEs with standard errors too large for meaningful analysis (15,19). Other documented sources of LE bias include contextual factors such as large nursing home populations, which skew distribution of local population structures (15,19).
These findings hold promise for generating local LEs for most American populations. With average population sizes of 4,000 and a general range of 1,200 to 8,000 (20), census tracts are similar in size to United Kingdom wards. Also, US Census data on nursing home populations and other group quarters is readily available (21).
Demonstrated Utility of Local Life Expectancy at Birth
Government agencies in England, Wales, Greece, and Australian New South Wales have used local LE for many public health applications, including identifying and tracking measurable reductions in health disparities (11,22), evaluating intervention effectiveness (14), and planning and funding local health services (14). Local LE has also been used to explore contributions of socioeconomic and environmental conditions to population health. For example, researchers exploring LE disparities in England and Wales reported the most important determinant to be “material poverty,” which is further influenced by sociodemographics, housing quality, and local economic policies (23,24).
Maps of LE help drive actions. Mapped LE inequalities between England’s northern and southern local authorities generated widespread media interest and catalyzed creation of Health Equity North, a collaboration of northern councils, the volunteer sector, National Health Service (NHS), and academia. Subsequent independent inquiry into root causes spurred national policy changes and increased community-centered investments focused on economic growth to reduce poverty; early childhood investments; transfer of authority and resources to local governments; and NHS services expanded beyond health care to address social determinants such as poverty, housing, education, and unemployment (25). For example, NHS partnered with Public Health England and others to fund 10 Healthy New Towns pilot sites, where 200,000 new housing units constructed in health-promoting neighborhoods will be monitored and evaluated for health effects (26).
In the United States, LE maps catalyzed local initiatives by highlighting disparities of up to 25 years across nearby neighborhoods in metropolitan areas including Oakland, California; Chicago, Illinois; Los Angeles, California; and New Orleans, Louisiana (9,10,27,28). Case examples from the Los Angeles County Department of Public Health (LACDPH) and Public Health–Seattle & King County (PHSKC) provide additional evidence of the utility of local LE maps.
Life expectancy at birth in Los Angeles County
In 2009, LACDPH examined LE disparities in the county. Although LE had increased steadily since 1991, large disparities were observed, including a nearly 18-year difference between black males (69.4 years) and Asian/Pacific Islander females (86.9 years). LACDPH recognized that actions addressing the underlying social and environmental health determinants were needed to reduce these disparities and advance equity. Partnerships with cities and unincorporated communities were established, and maps examining LE at matching geographic levels were created to increase engagement.
The adjusted Chiang II method was used to calculate single-year LE for 103 cities and unincorporated communities with populations greater than 15,000 (11,29). The Economic Hardship Index (EHI) was used to examine the relationship between LE and community-level social and economic conditions across communities (29). The EHI is a composite of 6 indicators (crowded housing, poverty, unemployment, educational attainment, population dependency, and income level) that provides a more complete picture of neighborhood conditions than any individual measure. The strong inverse relationship between the EHI score and LE prompted LACDPH to publish a report that ranks cities and communities by LE and economic hardship that was broadly disseminated via press releases and in print and electronic form to city mayors, council members, planners, and representatives from other health-related sectors such as education, housing, transportation, and business (27).
The report received substantial coverage in local, national, and international media and on local websites and blogs. Resulting increased awareness of the connection between social issues and health led to reframed city and community discussions around root causes of health and increased community engagement and motivation to act. For example, the report provided justification for a 2015 formal amendment to the Los Angeles General Plan, elevating health as a priority for the city’s future expansion and development. The amended plan includes a policy vision and measureable objectives for creating healthier communities through increased affordable housing, cleaner environments, and safer neighborhoods (30). Finally, the report strengthened LACDPH’s engagement with city and community leaders, education, business, and other nonhealth sectors and raised awareness of the importance of a Health-in-All-Policies approach, which considers the health implications of non-health–sector policies (31).
Life expectancy at birth in Seattle–King County
PHSKC staff calculated LE for King County using the adjusted Chiang II method (13) and 2012 mortality data. LE in King County (81.2 years) was substantially longer than LE in the United States. However, pronounced disparities across race/ethnicity and subregions were evident, so PHSKC staff examined census tract–level LE. In 2010, King County’s 398 tracts averaged 4,800 (range, 1,286–11,056) people. PHSKC used geocoded mortality data from 2008 through 2012 assigned to census tracts and locally generated population estimates to generate LE data. After suppressing cells with statistically unreliable estimates, results showed an LE gap of approximately 24 years between the shortest-lived and longest-lived tracts (Figure 1).
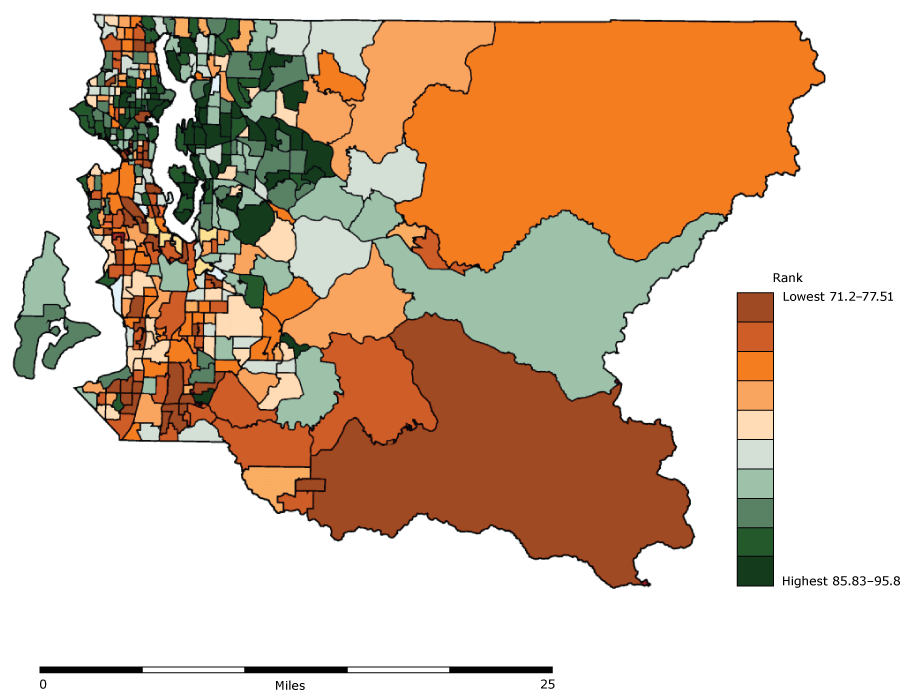
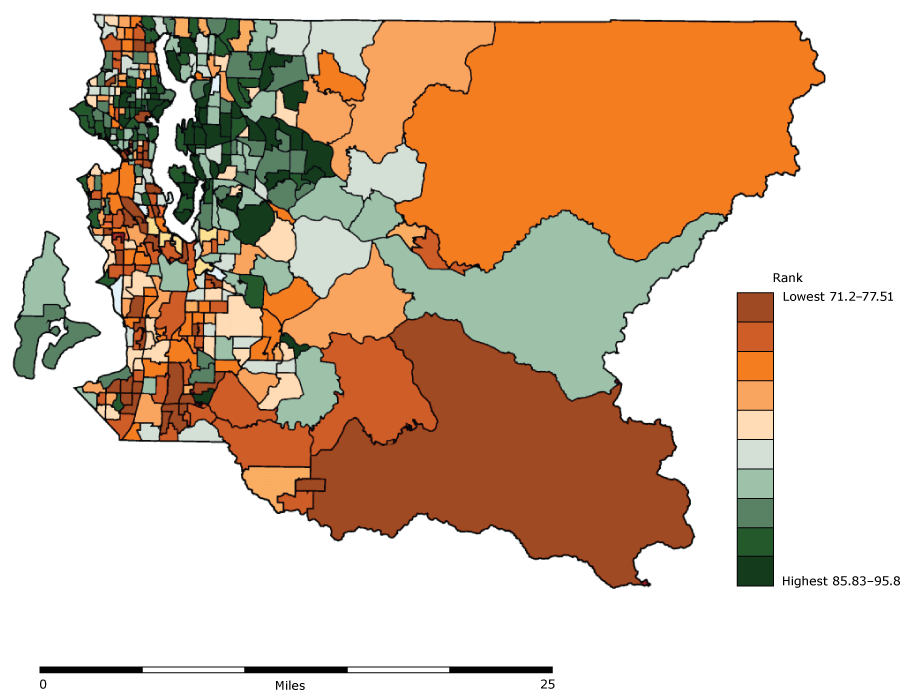
Figure 1.
Estimated life expectancy at birth by census tract in King County, Washington, based on 2008–2012 mortality data. [A text description of this figure is also available.]
Estimated life expectancy at birth by census tract in King County, Washington, based on 2008–2012 mortality data. [A text description of this figure is also available.]
PHSKC then used a Bayesian hierarchical model to generate mapped small-area estimates of modifiable risk factors such as adult obesity, smoking, adverse childhood experiences, preventable hospitalizations, poor housing conditions, high unemployment, low income, and adult frequent mental distress (32). Strikingly similar spatial patterns of disparities in LE and risk factors led to identification of potential communities for engagement; catalyzed an ongoing partnership between PHSKC, the Department of Community and Human Services, Seattle Foundation, and Living Cities; and led to formation of the Communities of Opportunity (COO) (33). COO focuses on improving equity in communities through system-level and policy-level solutions led by or engaging the local community. The COO collective impact framework includes community-identified achievement goals with identified indicators to measure progress. Desired results are that all people thrive economically; have quality, affordable housing; are healthy; and are connected to the community. To date, more than 90 community residents and 45 community organizations and their leaders have codesigned solutions (33).
Resources for Calculating Sub-County Life Expectancy at Birth
In September 2014, the Centers for Disease Control and Prevention (CDC) and the Council of State and Territorial Epidemiologists (CSTE) initiated the multiyear Sub-County Assessment of Life Expectancy (SCALE) project. The goal of SCALE Phase I, which ended in June 2015, was to identify appropriate methods for calculating actionable sub-county LE and develop easy-to-use resources designed to assist other health departments. For LE to be considered actionable, the method needed to produce accurate estimates for most of the jurisdiction’s populations with standard errors and confidence intervals narrow enough to permit identification of areas with significantly higher or significantly lower LE values.
Phase I participants included a CDC senior scientist, an external evaluator, and scientists from LACDPH and PHSKC, recruited on the basis of their previous experience. Additionally, scientists from 6 state health departments (Florida, Maine, Massachusetts, New York, Washington, and Wisconsin) were invited to participate because they varied in size and resources, their jurisdictions represented diverse geographies and populations, and they had experience examining relationships between small-area health and environmental indicators through an initiative of the National Environmental Public Health Tracking Network (EPHTN).
Phase I participant activities included a literature review to identify feasible methods, successful case studies, and gold-standard parameters. After each jurisdiction independently tested various approaches, a consensus was reached to adopt the adjusted Chiang II method and associated software developed by the South East Public Health Observatory (34). Phase I participants also developed a draft guidance document (Guide) clarifying methodological decision points (eg, age categories, addressing zero cells, minimum population sizes) and sharing lessons learned. SCALE Phase I and subsequent activities are described in Table 1.
The initial objective was standardized calculation of census-tract LE estimates using 5 years of death data (2008–2012) and 2010 census or local population estimates. Because SCALE is a user-driven initiative with a primary goal of supporting local actions, participants were encouraged to adapt the proposed objective and methods to meet their unique needs.
To evaluate feasibility of generating sub-county LE, interviews with each jurisdiction were conducted using questions designed to answer the following questions:
- What resources are required for health departments with varying resources and diverse populations to calculate actionable sub-county LE for the majority of their jurisdiction?
- What methodological and data challenges were encountered and how were they addressed?
SCALE Phase 1 Results and Lessons Learned
All jurisdictions reported successful calculation of actionable LE for most sub-county areas in less than 1.5 years, with 7 of the 8 participating jurisdictions completing calculations in less than 1 year.
Characteristics of participating jurisdictions and LE calculation approaches
Table 2 identifies characteristics of participating health departments and Table 3 describes the various LE approaches. Participating jurisdictions varied greatly on total expenditures, staffing, and total population size. Annual state health department expenditures for 2011 ranged from $108.08 million to $2.16 billion, and staffing ranged from 387 to 15,026 full-time equivalent employees (35). The 2016 Census population estimate for Maine of 1.3 million was smaller than those of the 2 county jurisdictions and approximately 16 times smaller than the estimated 20.6 million Florida residents (36).
Florida, Massachusetts, New York State, Washington, and PHSKC successfully calculated census tract–level LE with standard errors of less than 2 years for most of their populations using 5 years of data. All but one met the recommended minimum population size of 1,000 residents achieving 5,000 person-years-at-risk. Florida results included census tracts with the smallest and largest populations, 672 and 33,041 residents, respectively. Smaller and sparser Maine populations (37) required 10 years of data to generate LE with acceptable standard errors for most of their populations at the Minor Civil Divisions (MCDs) level. MCD is a US census bureau term for primary governmental divisions of a county such as townships. Wisconsin also required 10 years of data to calculate actionable LE at the zip code level. Aggregating data over time increases precision; however, the resulting LE may not reflect current conditions and increases the risk of numerator and denominator mismatch, which can bias standard errors (11,13,18). LACDPH chose to calculate single-year LE for areas with populations greater than 15,000.
Data challenges
Population and mortality data were readily available; however, some data sets were unsuitable or required additional manipulation. Florida explored the feasibility of calculating LE for inter-census periods, using American Community Survey (ACS) data. ACS data lacked population counts for the ideal age-intervals for calculating LE (<1 year and 1–4 year categories vs 0–4 years) and had high margins of error at the census tract level. As a result, LE estimates generated by using ACS data varied substantially from LE estimates generated by using 2010 Census data for the same sub-county area (46 years vs 65 years, respectively).
Erroneous and missing mortality data in some jurisdictions increased time and resource requirements. Special record requests were often necessary for residents dying in neighboring state jurisdictions. In Maine, mortality data lacked addresses before 2011; therefore, town of residence was used to assign deaths to MCDs. In New York State, mortality records required geocoding using varied batch and iterative techniques. Hospital records were used to correct incomplete or inaccurate address information. Geographic imputation techniques using age, race/ethnicity, town, and zip code were used to geocode remaining cases with missing addresses. Ultimately, census tracts were assigned to 99.97% of mortality records. However, these labor-intensive methods extended the project by several months. An article describing the New York State methods is under development.
Small number issues
Each jurisdiction included areas with populations too small to meet the recommended 5,000 person-years-at-risk. Florida, Massachusetts, PHSKC, Washington, and Wisconsin suppressed all LE values with standard errors greater than 2. Florida also suppressed improbable LE values of less than 66 years. The percentage of suppressed sub-county areas ranged from 3% to approximately 15%.
Before calculating LE, New York State excluded 18 census tracts that had no people and consisted of bodies of water, airports, and an uninhabited island. After exploratory analyses, tracts where more than 50% of the population lived in group quarters were also excluded. Consistent with the effects of nursing homes on LE values (16), improbable LE values were generated for tracts with large prison, military base, or college populations. Approximately 2.6% census tracts were ultimately excluded. Additionally, a New York State Geographic Aggregation Tool (38) was used to aggregate several neighboring census tracts until all had a minimum of 60 deaths and standard errors of less than 2 years.
Maine conducted exploratory analyses examining the effect of using a minimum standard error of 2 versus a standard error of 3 years, minimum number of deaths (>60), and minimum denominator (5,000 person-years). Depending on the rule(s), between 28% (standard error <3) and 46% (standard error <2 or deaths >60) of MCDs needed to be suppressed. Ultimately, Maine aggregated 10 years of data and several adjacent areas using the Geographic Aggregation Tool (38) until LE for all MCDs had a standard error of less than 2 years.
Discussion
America’s lagging health status and persistent local disparities warrant bold actions that address all determinants of health, including social and environmental factors. Identifying and quantifying local disparities is a necessary first step for selecting, implementing, and documenting the impact of interventions (9,39). LACDPH and PHSKC and other case studies document the use of sub-county LE for quantifying disparities and catalyzing multisector actions (26,31,33).
Many LE methodological challenges were identified, such as small number issues, missing and erroneous data, and lack of suitable population data for noncensus years. Small, sparse populations in 2 jurisdictions prohibited the calculation of census tract–level LE using 5 years of data. All jurisdictions included areas requiring suppression of LE data or additional temporal or geographical aggregation. However, these solutions may not be as effective for even smaller or more sparsely populated jurisdictions. Finally, LE does not fully reflect health status or other dimensions of well-being through the life course (40).
As part of SCALE Phase II, initiated in June 2015, 17 additional health departments successfully calculated sub-county LE, pilot tested the Guide, and provided feedback on its usability and utility (Figure 2). In September 2016, CSTE launched a SCALE website (www.cste.org/page/SCALE/Sub-County-Assessment-of-Life-Expectancy-SCALE-Project.htm), which includes version 1.0 of the updated Guide and other user-friendly resources. A joint SCALE and EPHTN workshop was held in October 2016, with objectives of prioritizing future collective activities and supporting sub-county LE calculation by the 20 EPHTN grantees not previously engaged in SCALE.
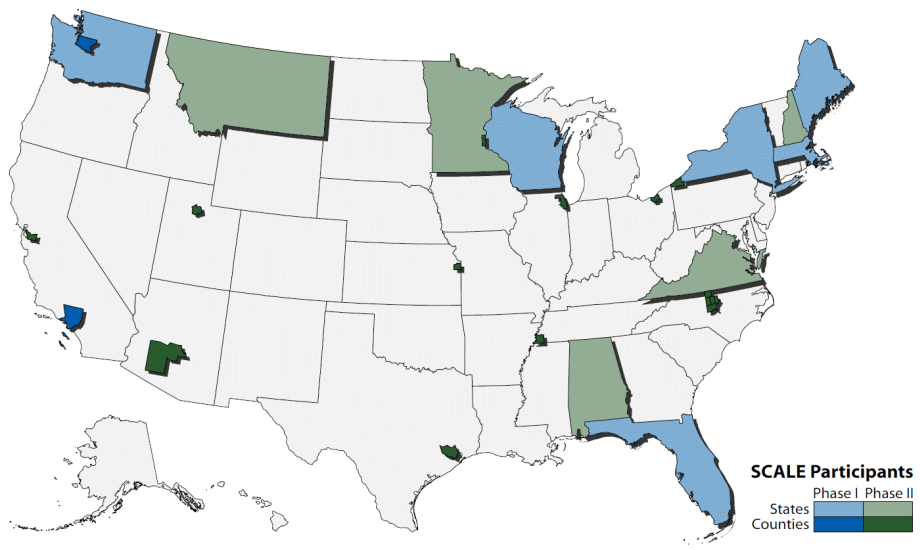
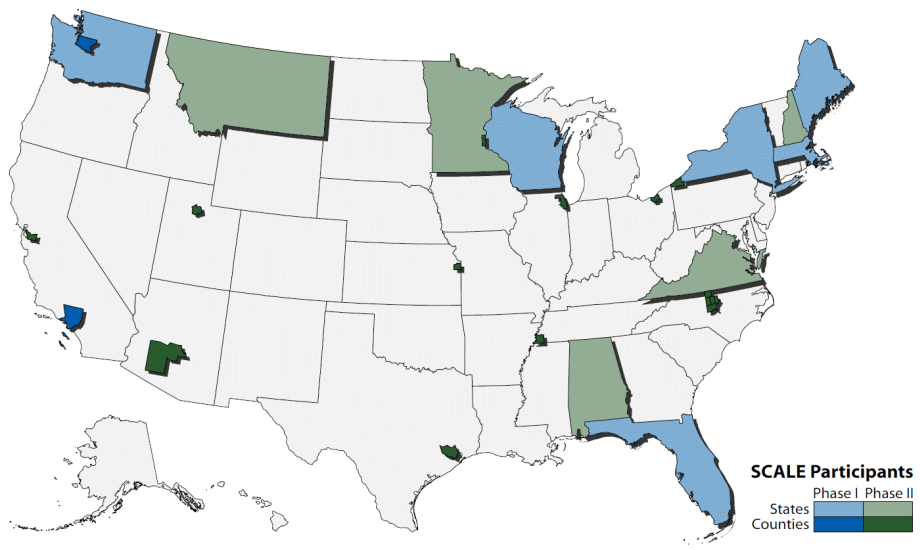
Figure 2.
United States map identifying health department jurisdictions of SCALE Phase I and II participants. Abbreviation: SCALE, Sub-County Assessment of Life Expectancy. [A text description of this figure is also available.]
United States map identifying health department jurisdictions of SCALE Phase I and II participants. Abbreviation: SCALE, Sub-County Assessment of Life Expectancy. [A text description of this figure is also available.]
Prioritized future activities include identifying key local social determinant and health indicators for co-release with LE estimates; assessing feasibility of generating summary population measures that better reflect overall health, including health-adjusted life expectancy; identifying LE visualization and messaging best practices; and evaluating the utility of local LE for monitoring and evaluating the health effects of local policies and programs.
Current and planned SCALE resources directly respond to calls for locally relevant data capable of identifying geographic disparities, catalyzing multisector actions, and evaluating the effects of interventions designed to improve population health and advance equity. Lessons learned and user-friendly resources are provided to help accelerate widespread scaling of these efforts.
Acknowledgments
The authors acknowledge the contribution and dedication of the SCALE Phase I Working Group members: Melissa Jordan (Florida Department of Health), Kristina Kintziger (formerly with Florida Department of Health), Douglas Done (New York State Department of Health), Tabassum Insaf (New York State Department of Health), Thomas Talbot (New York State Department of Health), Jessica Bonthius (Maine Department of Health and Human Services), Chris Paulu (Maine Department of Health and Human Services), Alicia Fraser (Massachusetts Department of Public Health), Robert Knorr (Massachusetts Department of Public Health), Glen Patrick (Washington State Department of Health), Henry Anderson (formerly with Wisconsin Division of Public Health), and Jessica Wurster (CSTE).
This publication was supported by Cooperative Agreement no. 1U38OT000143 awarded to CSTE by CDC. The findings and conclusions in this manuscript are those of the authors and do not necessarily represent the official position of CDC.
Author Information
Corresponding Author: Vickie L. Boothe, MPH, Division of Public Health Information Dissemination, Center for Surveillance, Epidemiology, and Laboratory Services, Centers for Disease Control and Prevention, 1600 Clifton Rd NE, Mail Stop E69, Atlanta, GA 30333. Telephone: 404-498-2826. Email: veb6@cdc.gov.
Author Affiliations: 1Centers for Disease Control and Prevention, Atlanta, Georgia. 2Claremont Graduate University, Los Angeles, California. 3Seattle and King County Public Health, Seattle, Washington. 4Los Angeles County Department of Public Health, Los Angeles, California.
References
- Centers for Disease Control and Prevention (CDC). Ten great public health achievements—United States, 1900–1999. MMWR Morb Mortal Wkly Rep 1999;48(12):241–3. PubMed
- Kochanek KD, Murphy SL, Xu J, Tejada-Vera B. Deaths: final data for 2014. Natl Vital Stat Rep 2016;65(4):1–122. PubMed
- Xu JQ, Murphy SL, Kochanek KD, Arias E. Mortality in the United States, 2015. NCHS data brief, no 267. Hyattsville (MD): National Center for Health Statistics; 2016.
- Institute of Medicine, National Research Council. US health in international perspective: shorter lives, poorer health. Washington (DC): The National Academies Press; 2013.
- Wang H, Schumacher AE, Levitz CE, Mokdad AH, Murray CJ. Left behind: widening disparities for males and females in US county life expectancy, 1985–2010. Popul Health Metr 2013;11(1):8–8. CrossRef PubMed
- Cullen MR, Cummins C, Fuchs VR. Geographic and racial variation in premature mortality in the U.S.: analyzing the disparities. PLoS One 2012;7(4):e32930. CrossRef PubMed
- DeSalvo KB, O’Carroll PW, Koo D, Auerbach JM, Monroe JA. Public Health 3.0: time for an upgrade. Am J Public Health 2016;106(4):621–2. CrossRef PubMed
- Shah SN, Russo ET, Earl TR, Kuo T. Measuring and monitoring progress toward health equity: local challenges for public health. Prev Chronic Dis 2014;11(9):E159. PubMed
- Hunt BR, Tran G, Whitman S. Life expectancy varies in local communities in Chicago: racial and spatial disparities and correlates. J Racial Ethn Health Disparities 2015;2(4):425–33.CrossRef PubMed
- Schaff K, Desautels A, Flournoy R, Carson K, Drenick T, Fujii D, et al. Addressing the social determinants of health through the Alameda County, California, place matters policy initiative. Public Health Rep 2013;128(Suppl 3):48–53. CrossRef PubMed
- Eayres D, Williams ES. Evaluation of methodologies for small area life expectancy estimation. J Epidemiol Community Health 2004;58(3):243–9. CrossRef PubMed
- Auger N, Feuillet P, Martel S, Lo E, Barry AD, Harper S. Mortality inequality in populations with equal life expectancy: Arriaga’s decomposition method in SAS, Stata, and Excel. Ann Epidemiol 2014;24(8):575–80, 580.e1. CrossRef PubMed
- Silcocks PB, Jenner DA, Reza R. Life expectancy as a summary of mortality in a population: statistical considerations and suitability for use by health authorities. J Epidemiol Community Health 2001;55(1):38–43. CrossRef PubMed
- Stephens AS, Purdie S, Yang B, Moore H. Life expectancy estimation in small administrative areas with non-uniform population sizes: application to Australian New South Wales local government areas. BMJ Open 2013;3(12):e003710. CrossRef PubMed
- Jonker MF, van Lenthe FJ, Congdon PD, Donkers B, Burdorf A, Mackenbach JP. Comparison of Bayesian random-effects and traditional life expectancy estimations in small-area applications. Am J Epidemiol 2012;176(10):929–37. CrossRef PubMed
- Zhang X, Holt JB, Yun S, Lu H, Greenlund KJ, Croft JB. Validation of multilevel regression and poststratification methodology for small area estimation of health indicators from the Behavioral Risk Factor Surveillance System. Am J Epidemiol 2015;182(2):127–37. CrossRef PubMed
- Miller TM, Abdel-Maksoud MF, Crane LA, Marcus AC, Byers TE. Effects of social approval bias on self-reported fruit and vegetable consumption: a randomized controlled trial. Nutr J 2008;7(1):18. CrossRef PubMed
- Toson B, Baker A. Life expectancy at birth: methodological options for small populations. Norwich (UK): Office for National Statistics; 2003.
- Scherbov S, Ediev D. Significance of life table estimates for small populations: simulation-based study of estimation errors. Demogr Res 2011;24(22):527–50. CrossRef
- Krieger N, Chen JT, Waterman PD, Soobader MJ, Subramanian SV, Carson R. Choosing area based socioeconomic measures to monitor social inequalities in low birth weight and childhood lead poisoning: The Public Health Disparities Geocoding Project (US). J Epidemiol Community Health 2003;57(3):186–99. CrossRef PubMed
- National Research Council, Committee on National Statistics, Division of Behavioral and Social Sciences and Education. Small populations, large effects: improving the measurement of the group quarters population in the American Community Survey. In: Panel on statistical methods for measuring the group quarters population in the American Community Survey, Paul R. Voss, Krisztina Marton, editors. Washington (DC): The National Academies Press; 2012.
- Tsimbos C, Kalogirou S, Verropoulou G. Estimating spatial differentials in life expectancy in Greece at local authority level. Popul Space Place 2014;20(7):646–63. CrossRef
- Woods LM, Rachet B, Riga M, Stone N, Shah A, Coleman MP. Geographical variation in life expectancy at birth in England and Wales is largely explained by deprivation. J Epidemiol Community Health 2005;59(2):115–20. CrossRef PubMed
- Doran TF, Drever F, Whitehead M. Health underachievement and overachievement in English local authorities. J Epidemiol Community Health 2996;60(8):686–93.
- Johnstone P. Health, equity and the north of England: a case study on a new approach. Br Med Bull 2015;116(1):29–41. PubMed
- Norman H, McDonnell D. The NHS Healthy New Towns programme. Perspect Public Health 2017;137(1):29–30. CrossRef PubMed
- Life expectancy in Los Angeles County: how long do we live and why? A cities and communities health report. Los Angeles (CA): Los Angeles County Department of Public Health; 2010. http://www.publichealth.lacounty.gov/epi/docs/life%20expectancy%20final_web.pdf. Accessed October 11, 2016.
- Place Matters for Health in Orleans Parish: ensuring opportunities for good health for all. A report on health inequities in Orleans Parish, Louisiana. Joint Center for Political and Economic Studies; 2012. http://jointcenter.org/research/place-matters-health-orleans-parish-ensuring-opportunities-good-health-all. Accessed October 11, 2016.
- Montiel LM, Nathan RP, Wright DJ. An update on urban hardship. Albany (NY): Nelson A. Rockefeller Institute of Government; 2004. http://rockinst.org/pdf/cities_and_neighborhoods/2004-08-an_update_on_urban_hardship.pdf.
- Plan for a Healthy Los Angeles. A health and wellness element of the general plan. http://planning.lacity.org/cwd/gnlpln/PlanforHealthyLA.pdf. Accessed June 25, 2017.
- Fielding JE, Teutsch SM, Caldwell S. Public health practice: what works. New York (NY): Oxford University Press; 2012.
- Song L, Mercer L, Wakefield J, Laurent A, Solet D. Using small-area estimation to calculate the prevalence of smoking by subcounty geographic areas in King County, Washington, Behavioral Risk Factor Surveillance System, 2009–2013. Prev Chronic Dis 2016;13:150536. CrossRef PubMed
- Communities of opportunity. Public Health Seattle King County; 2016. http://www.kingcounty.gov/elected/executive/health-human-services-transformation/coo.aspx. Accessed June 25, 2017.
- Life expectancy calculator: LA and ward level; 2004. http://webarchive.nationalarchives.gov.uk/20160701122411/http://www.sepho.org.uk/viewResource.aspx?id=8943. Accessed June 25, 2017.
- Association of State and Territorial Health Officials. ASTHO profile of state public health, vol. 3. Washington (DC): Association of State and Territorial Health Officials; 2014.
- US Census Bureau. Quick facts. http://www.census.gov/quickfacts/table/PST045216/00. Accessed June 25, 2017.
- US Census Bureau. Population density by state, US Census Bureau. http://www.StateMaster.com. Accessed November 29, 2017.
- Talbot TO, LaSelva GD. Geographic aggregation tool for R, version 1.33. Albany (NY): New York State Health Department; 2015. http://www.albany.edu/faculty/ttalbot/GAT/. Accessed June 25, 2017.
- Clarke CA, Miller T, Chang ET, Yin D, Cockburn M, Gomez SL. Racial and social class gradients in life expectancy in contemporary California. Soc Sci Med 2010;70(9):1373–80. CrossRefPubMed
- Marmot M. Fair society, healthy lives: strategic review of health inequalities in England post 2010. London (UK): Institute of Health Equity; 2010.
No hay comentarios:
Publicar un comentario